2020-10-06
Python İle Basit Bir Derin Öğrenme Uygulaması
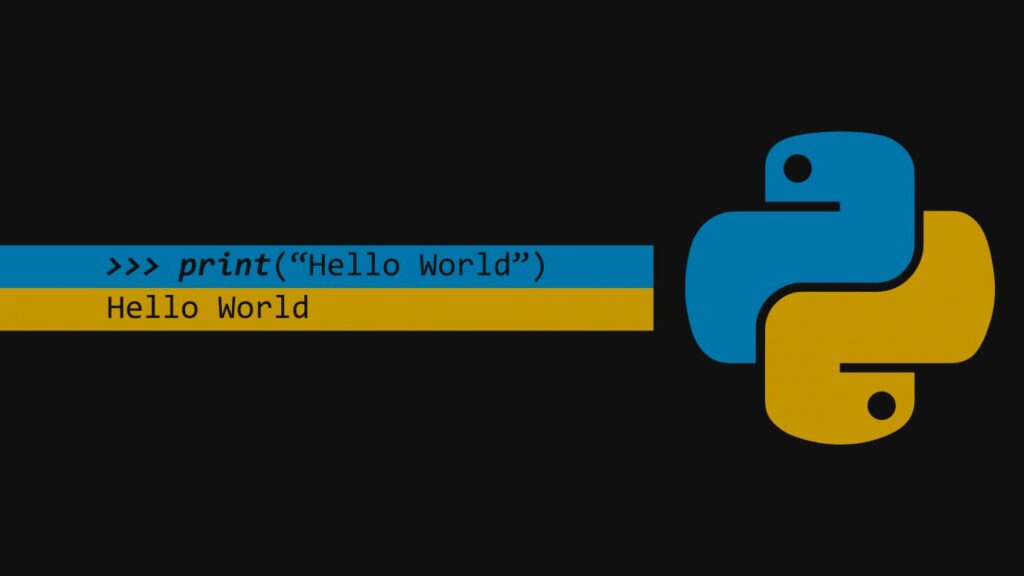
Python ile veri bilimi ve makine öğrenmesi konusunda çalışmak istiyorsak işe NumPy ve Pandas ile başlamamız gerekiyor.
This file contains bidirectional Unicode text that may be interpreted or compiled differently than what appears below. To review, open the file in an editor that reveals hidden Unicode characters.
Learn more about bidirectional Unicode characters
import numpy as np | |
# sigmoid function | |
def nonlin(x, deriv=False): | |
if (deriv == True): | |
return x * (1 – x) | |
return 1 / (1 + np.exp(-x)) | |
# input dataset | |
X = np.array([[1, 0, 1], | |
[1, 1, 1], | |
[0, 0, 1], | |
[0, 1, 1]]) | |
# output dataset | |
y = np.array([[0, 0, 1, 1]]).T | |
np.random.seed(1) | |
syn0 = 2 * np.random.random((3, 1)) – 1 | |
for iter in range(10000): | |
# forward propagation | |
l0 = X | |
l1 = nonlin(np.dot(l0, syn0)) | |
l1_error = y – l1 | |
# print(l1_error) | |
l1_delta = l1_error * nonlin(l1, True) | |
# print(l1_delta) | |
syn0 += np.dot(l0.T, l1_delta) | |
print("input") | |
print(X) | |
print("output") | |
print(y) | |
print("Output After Training:") | |
print(l1) |
Çok basit bir örnek uygulama ile başlayabiliriz. Bir giriş veri setimiz var, bir işlemden geçerek bir sonuç seti oluşuyor. Bunun algoritmasını tahmin etmeye çalışıyoruz.
Sigmoid fonksiyonu ile iterasyonu ayarlıyoruz. Bu temel bir yaklaşımdır.