DBSCAN: The Navigator of Geolocation Data
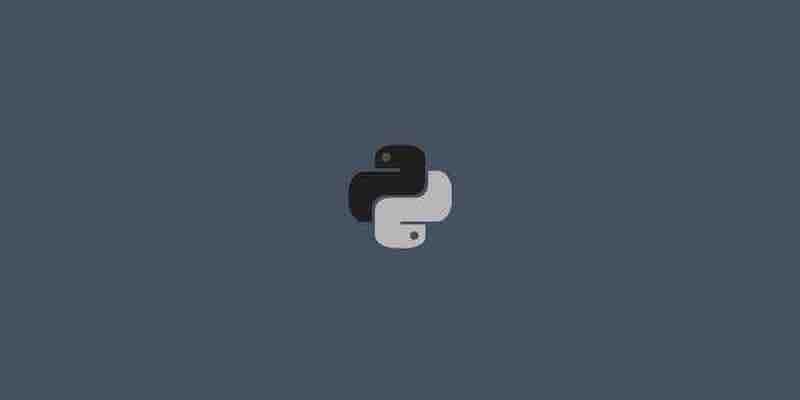
Why DBSCAN? What makes DBSCAN a strong choice for geolocation data?
No Need for a Compass | First and foremost, DBSCAN doesn’t require you to specify the number of clusters in advance. It’s like a seasoned sailor instinctively finding islands in the sea of data. This starkly contrasts with algorithms like K-means, which need a predefined number of clusters. |
Navigating the Storms | DBSCAN requires relatively less domain knowledge. You don’t need to know the number of clusters beforehand, which iIt’sten hard to determine for geolocation data. |
Flexible Boundaries | DBSCAN doesn’t force data into spherical clusters; it gracefully adapts to clusters of various shapes and sizes. Imagine a coastline that twists and turns – DBSCAN can follow these natural contours, making it ideal for geolocation data. |
Density: The Guiding Star | DBSCAN stands for Density-Based Spatial Clustering of Applications with Noise. It groups points that are closely packed based on a density criterion. In geolocation, this is invaluable. It allows the algorithm to find areas with densely packed locations, such as urban centers, and distinguish them from more sparse regions, like rural areas. |
Minimal Manual Steering | DBSCAN is robust to noise. In geolocation, not all data points are part of a meaningful cluster that doesn’t identifies these outliers and classifies them as noise, ensuring they don’t distort the overall picture. |
DBSCAN, with its ability to find clusters of arbitrary shapes, its robustness to noise, and its minimal requirements for manual input, emerges as a strong choice for clustering geolocation data. It’s like having an expert navigator for spatial data’s complex, winding routes.